Introduction
Before we start let’s first understand what is Data Transformation?
Data transformation is the process of converting data from its original format or structure into a format that is more appropriate for analysis, reporting, or further processing.
But what does this data transformation mean in Power BI?
Data transformation in Power BI refers to the process of modifying and refining raw data to make it more suitable for analysis and visualization. This process is performed in the Power Query Editor, a powerful tool within Power BI that allows users to clean, shape, and transform data. Here are key aspects of data transformation in Power BI:
Key Aspects of Data Transformation
Data Cleaning
- Removing Duplicates: Identifying and eliminating duplicate records to ensure data integrity.
- Handling Missing Values: Addressing null or missing values by filling them in, replacing them, or removing the affected records.
- Filtering Data: Removing unnecessary or irrelevant data based on specific criteria.
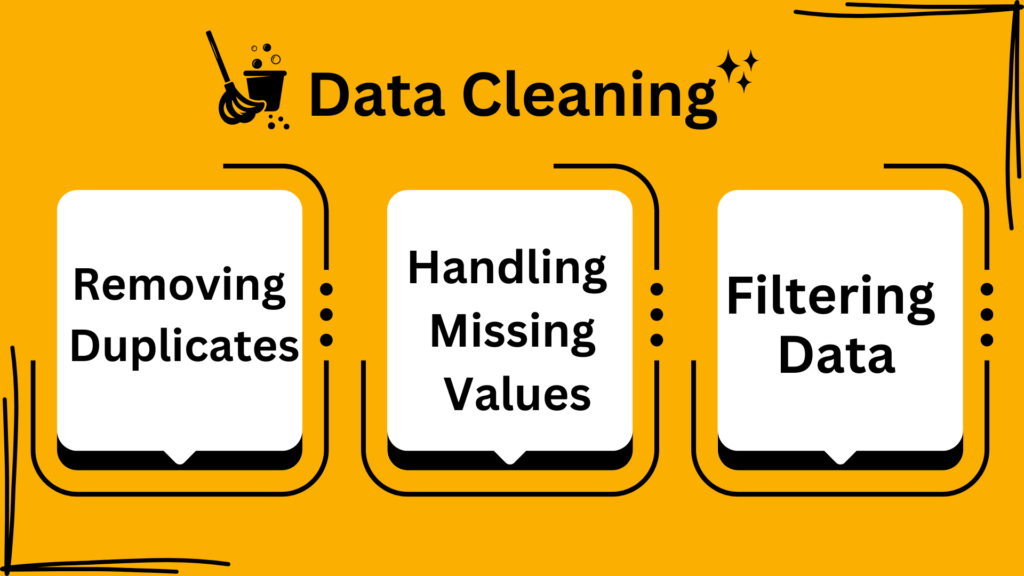
Data Shaping
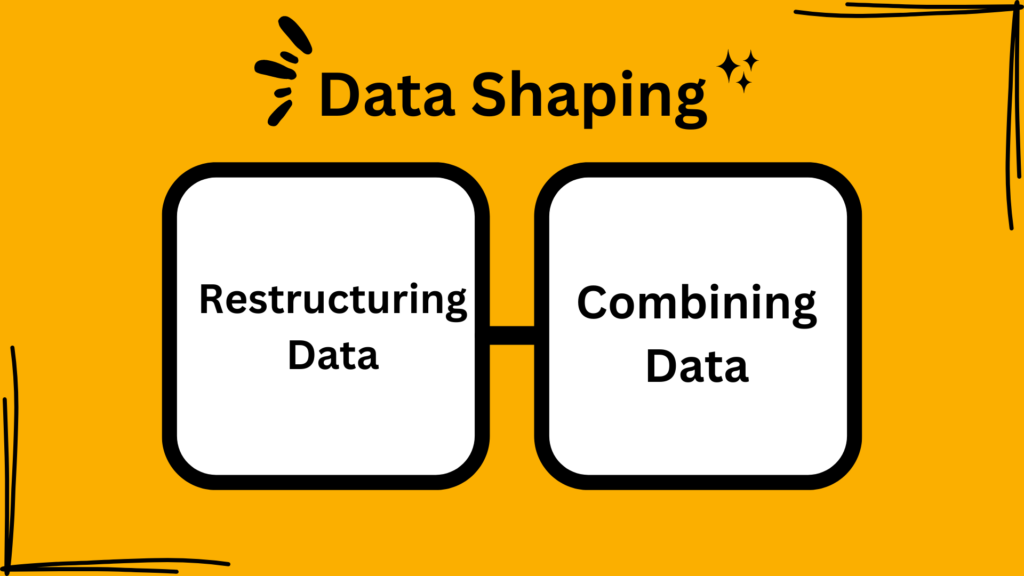
Data Enrichment
- Adding Calculations: Creating new columns or fields based on calculations or transformations of existing data.
- Aggregating Data: Summarizing data through functions like sum, average, count, etc., to provide higher-level insights.
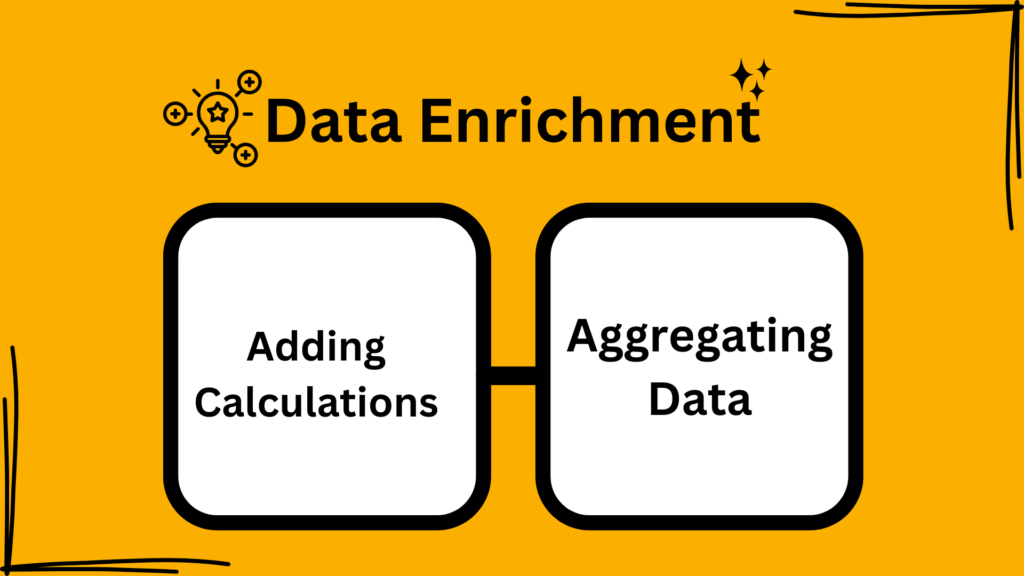
Data Type Conversion
- Standardizing Data Types: Ensuring data fields have consistent and appropriate data types (e.g., converting text to numeric or date formats).
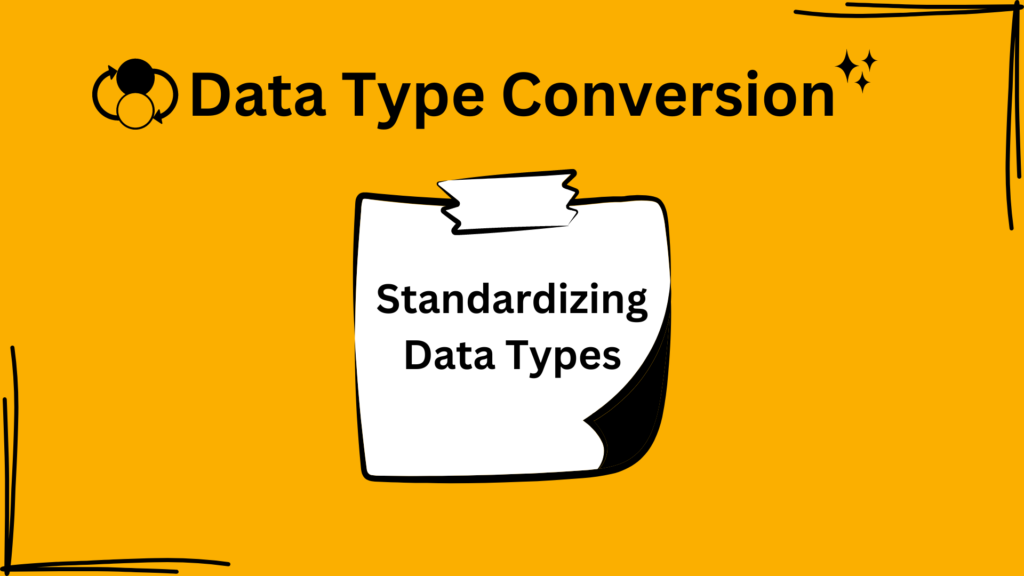
Column and Row Operations
- Splitting and Merging Columns: Dividing a column into multiple columns or combining several columns into one.
- Sorting and Reordering: Arranging data in a specific order or rearranging columns for better organization.
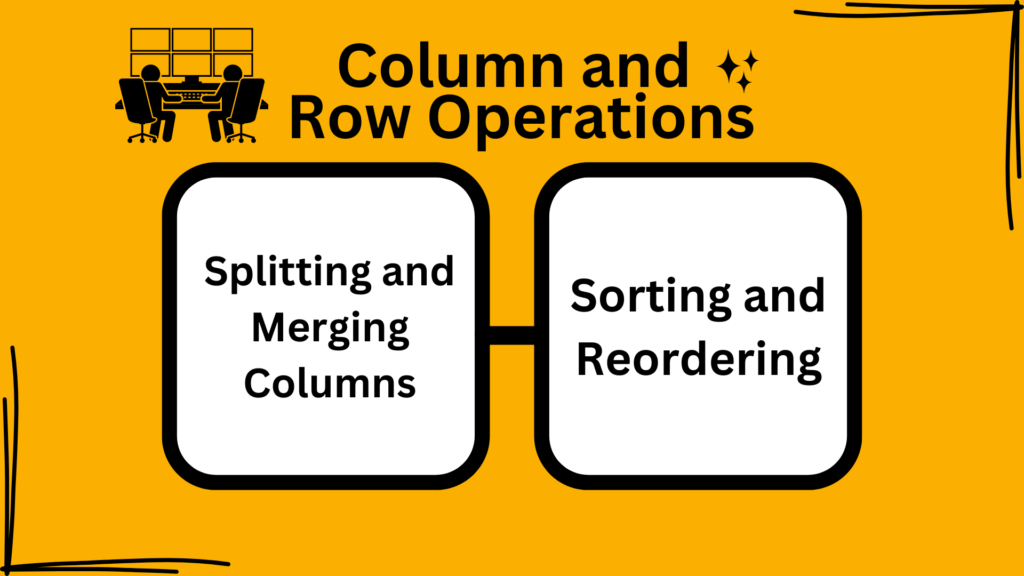
Data Validation
- Ensuring Accuracy: Checking data for errors, inconsistencies, or anomalies to maintain high data quality.
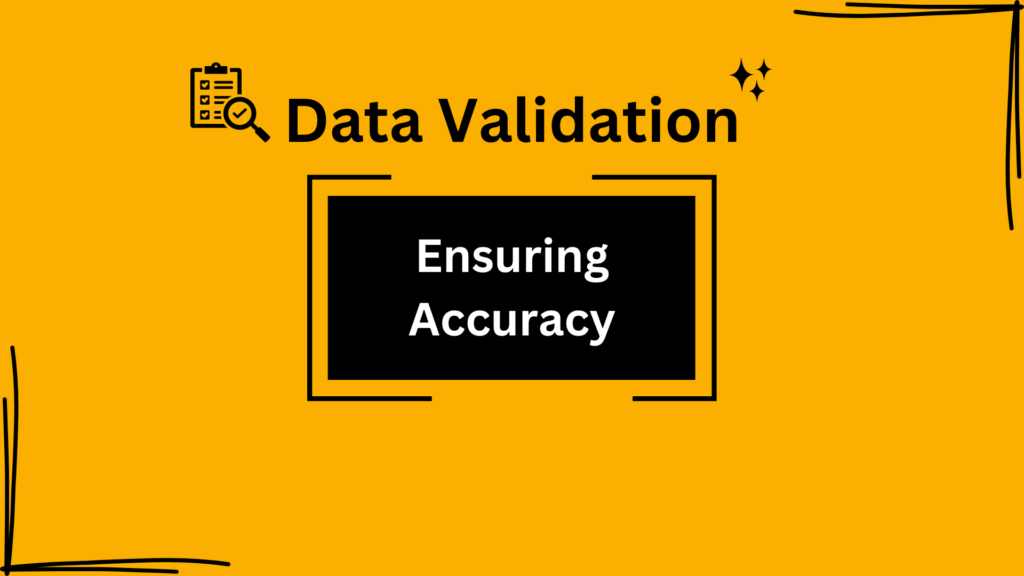
Examples of Data Transformation
- In Business Intelligence (BI) Tools: In tools like Power BI, data transformation involves importing raw data, cleaning it, and transforming it within the Power Query Editor before using it for reporting and analysis.
- In Databases: When loading data into a data warehouse, ETL (Extract, Transform, Load) processes transform data from operational databases into a format suitable for analytical querying.
- In Data Integration: When integrating data from different sources, transformation is necessary to ensure consistency and compatibility across the combined dataset.
Practical Steps in Data Transformation
- Extract: Retrieve raw data from various sources (databases, spreadsheets, APIs, etc.).
- Transform: Apply cleaning, shaping, and enrichment techniques to prepare the data.
- Load: Load the transformed data into the target system (e.g., a data warehouse, BI tool, or database).
Importance of Data Transformation
- Improves Data Quality: Ensures that data is accurate, consistent, and reliable.
- Enhances Usability: Converts data into a format that is easier to analyze and use for decision-making.
- Facilitates Integration: Makes it possible to combine data from diverse sources into a unified dataset.
- Supports Advanced Analytics: Prepares data for more complex analysis, such as machine learning or predictive modeling.
Conclusion
Data transformation is a critical step in the data processing workflow, essential for preparing data for analysis and decision-making. By converting raw data into a clean, structured, and enriched format, organizations can unlock valuable insights and make informed decisions based on accurate and relevant information.
Frequently Asked Questions
Data transformation is the process of converting data from its original format into a format that is more suitable for analysis, reporting, or further processing. This includes cleaning, shaping, and enriching the data to make it more usable and valuable.
Data transformation is important because it improves data quality, enhances usability, facilitates integration, and supports advanced analytics. It ensures that data is accurate, consistent, and in a format suitable for decision-making and analysis.
The common steps in data transformation include:
Data cleaning (removing duplicates, handling missing values, filtering data)
Data shaping (restructuring, combining data)
Data enrichment (adding calculations, aggregating data)
Data type conversion (standardizing data types)
Column and row operations (splitting, merging, sorting, reordering)